NLP COLLOQUIUM hosted by Prof. Dr. Lucie Flek
Hosted by Prof. Dr. Lucie Flek from the University of Bonn, our NLP Colloquium brings together researchers from diverse fields and institutions across the globe. We provide a platform for in-depth presentations on the latest advancements in Natural Language Processing.
Explore our schedule of upcoming talks and become a part of the conversation and community. Register and join us in person or online.
PAST EVENTS
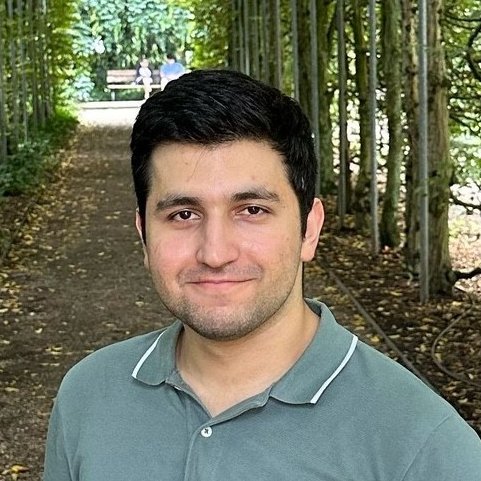
Guest Talk: NoLiMa: Long-Context Evaluation Beyond Literal Matching - 11.06.2025, 10:00 - 11:00
Speaker: Ali Modarressi (LMU Munich)
Abstract: Recent large language models (LLMs) support long contexts ranging from 128K to 1M tokens. A popular method for evaluating these capabilities is the needle-in-a-haystack (NIAH) test, which involves retrieving a "needle" (relevant information) from a "haystack" (long irrelevant context). Extensions of this approach include increasing distractors, fact chaining, and in-context reasoning. However, in these benchmarks, models can exploit existing literal matches between the needle and haystack to simplify the task. To address this, we introduce NoLiMa, a benchmark extending NIAH with a carefully designed needle set, where questions and needles have minimal lexical overlap, requiring models to infer latent associations to locate the needle within the haystack. We evaluate 12 popular LLMs that claim to support contexts of at least 128K tokens. While they perform well in short contexts (<1K), performance degrades significantly as context length increases. At 32K, for instance, 10 models drop below 50% of their strong short-length baselines. Even GPT-4o, one of the top-performing exceptions, experiences a reduction from an almost-perfect baseline of 99.3% to 69.7%. Our analysis suggests these declines stem from the increased difficulty the attention mechanism faces in longer contexts when literal matches are absent, making it harder to retrieve relevant information.
Bio: Ali is a third-year PhD student at the Center for Information and Language Processing (CIS) at LMU Munich, working under the supervision of Prof. Hinrich Schütze. His current research focuses on memory-augmented large language models, and, more broadly, on long-context language modeling and interactive language generation, and information extraction. His NLP journey began during his MSc, supervised by Mohammad Taher Pilehvar, where he worked on explainability methods and interpretability of pre-trained language models—an area that remains relevant to his current research, particularly in analyzing retrieval models and knowledge probing.
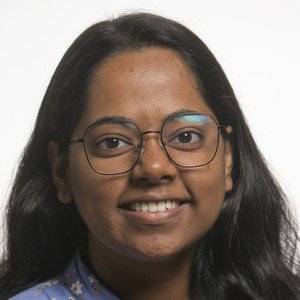
Guest Talk: Affective Traits of Natural Language - 24.04.2025, 12:15 - 13:45
Speaker DAAD AInet Fellows: Shivani Kumar (University of Michigan)
Abstract: Over the past decade, Natural Language Processing (NLP) has undergone a transformative journey, marked by profound changes, particularly in the development of Large Language Models (LLMs). While some applications of LLMs, such as dialogue agents, have become a common part of our daily lives, their underlying complexities can go unnoticed. This talk focuses on one key aspect of language comprehension—affects. Affective traits encompass factors such as emotions, humor, sarcasm, and moral values, all of which are essential for fully understanding what is being communicated. Our work examines these subtle elements, aiming to enhance the interpretative abilities of LLMs by deepening their understanding of these traits in language, contributing to more meaningful human-machine interactions.
Bio: Shivani Kumar is a Postdoctoral Research Fellow at the School of Information at University of Michigan. Her current work focuses on developing culturally enriched and morally refined language models. She earned her PhD from IIIT Delhi, India, where she studied conversational AI and focused on how people express themselves in dialogue – including emotions, humor, sarcasm, and individual speaking styles. Shivani’s future research goals involve exploring how LLMs manage rhetorical elements in conversations, particularly in the domains of ethos, pathos, and logos.
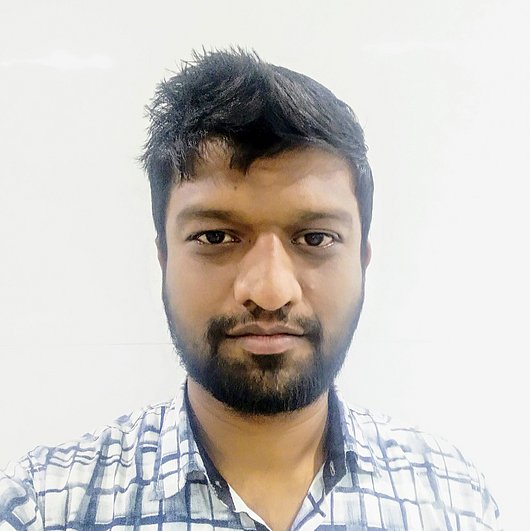
Guest Talk: Waking LLMs from CryoSleep with Continual Learning - 24.04.2025, 12:15 - 13:45
Speaker DAAD AInet Fellows: Yash Kumar Atri (University of Virginia)
Abstract: Large Language Models (LLMs) are often seen as powerful yet static entities, their knowledge frozen after training, disconnected from the ever-evolving world. In this talk, we will explore the challenge of updating these models without retraining them from scratch. We’ll examine current techniques such as fine-tuning, parameter-efficient methods (PEFT), Retrieval-Augmented Generation (RAG), and model editing approaches like Elastic Weight Consolidation (EWC), each with its own trade-offs in scalability, consistency, and memory retention.
But what comes next? Can LLMs evolve continuously, much like human learners? This talk will delve into the concept of incremental and continual learning for LLMs, why it’s challenging, what it entails, and how we might move toward systems that truly learn and adapt over time, without forgetting their past knowledge.
Bio: Yash Kumar is a Postdoctoral Research Associate at the University of Virginia, where his research focuses on model editing, continual learning, and neural reasoning. His work focuses on developing efficient methods for updating large language models (LLMs) to refine knowledge, minimize hallucinations, and enable continuous adaptation without catastrophic forgetting. Yash holds a Ph.D. in Computer Science and Engineering from IIIT Delhi, where his research focused on abstractive text summarization.Looking forward to your participation.
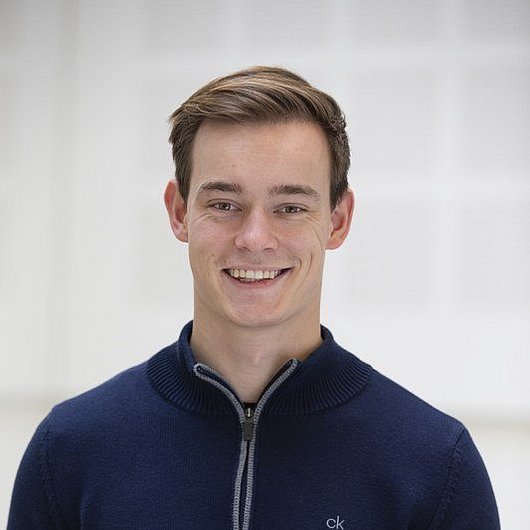
APRIL 2025
Guest Talk: Structured Summarization of German Clinical Dialogue in Orthopedy - 16.04.2025, 10:00 - 11:00
Speaker: Fabian Lechner (University of Marburg)
Abstract: The integration of machine learning, particularly large language models (LLMs), into medical applications offers great potential to conduct clinical documentation. This study explores the feasibility and effectiveness of generating structured medical letters exclusively from conversational data between physicians and patients. Using only local models such as the whisper speech-to-text models for transcription and local instance of phi-4 for summarization, we aim to automate the creation of clinical documentation while also generating free to use gold standard datasets for future research. The methodology involves recording 100 real-world physician-patient consultations in clinical settings, transcribing the conversations into text, and generating clinical letters using only local models. These outputs will be systematically evaluated by medical professionals for completeness, accuracy, and clarity against manually created letters. All data processing is conducted securely within the University Hospital Bonn’s infrastructure, ensuring compliance with GDPR and ethical standards. This project provides a novel framework for assessing the practical application of AI in clinical documentation, with implications for improving efficiency in healthcare workflows.
Bio: Fabian Lechner is a researcher at the Institute for Artificial Intelligence in Medicine and the Institute of Digital Medicine at Philipps University of Marburg. He holds degrees in Business Administration from Aachen and Business Informatics from Marburg. His master’s thesis with Prof. Flek focused on integrating large language models, such as ChatGPT and GPT-3, into medical processes. Since October 2022, Lechner has contributed to several publications, including studies on AI-driven decision support systems in oncology and the adoption of digital health applications among physicians.
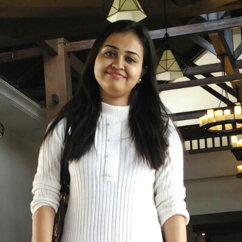
MARCH 2025
Guest Talk: Efficient Language Model Adaptation: Bridging the Gap with Limited Resources - 25.03.2025 15:30
Speaker DAAD AInet Fellows: Mohna Chakraborty (University of Michigan)
Abstract: Large language models (LLMs) have demonstrated remarkable capabilities, but their high computational costs and reliance on extensive labeled data limit their practical deployment in resource-constrained settings. This talk explores strategies for efficiently adapting and leveraging smaller, more deployable models while minimizing reliance on human annotations.
I will discuss research on overcoming key challenges in model adaptation, including mitigating sensitivity to prompt variations, improving label efficiency through weak supervision, and optimizing sample selection in low-resource scenarios. Additionally, I will present ongoing efforts to narrow the performance gap between small and large LLMs through knowledge distillation. By integrating insights from model evaluation, data selection, and training optimizations, this talk highlights practical methodologies for achieving competitive performance while working within computational and budgetary constraints.
Bio: I am a post-doctoral fellow at the University of Michigan (Michigan Institute for Data and AI in Society) under the guidance of Dr. David Jurgens and Dr. Lu Wang. I finished my Ph.D. in Computer Science from Iowa State University. I have worked as a Research Assistant in the Data Mining and Knowledge Lab under my advisor, Dr. Qi Li. I have also worked as a Data Science intern at The Home Depot, Epsilon, and a Data Analytics intern at Delaware North. My research interests are in the domain of data mining, natural language processing, and machine learning. Through my research, I have contributed several key methods in top conferences like PAKDD' 2025, SIAM' 2025, ACL' 2023, UAI' 2023, SIGKDD' 2022, ESEC/FSE'2021 and workshops like ICLR' 2025, WWW' 2025, PAKDD' 2025, RANLP'2021.
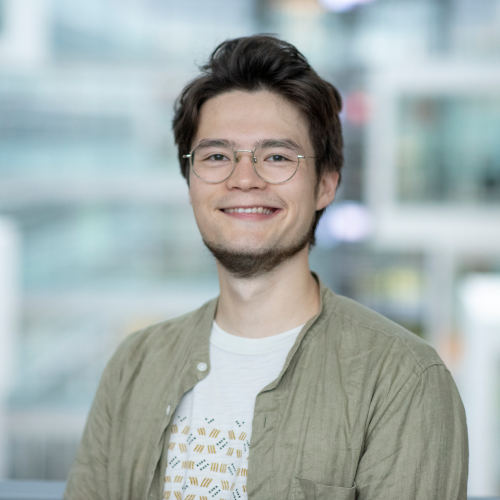
Guest Talk: Dynamic Personalization from Cross-model Consistencies - 18.3.2025 16:00
Speaker DAAD AInet Fellows: Maximilian Müller-Eberstein (IT University of Copenhagen)
Abstract: Scaling up Language Models has led to increasingly advanced capabilities for those who can afford to train them. In order to enable community-tailored models for the rest of us, we will examine cross-model consistencies in how LMs acquire their linguistic knowledge—from fundamental syntax and semantics up to higher-level pragmatic features, such as culture. By identifying these consistencies across different models, we highlight opportunities for how they can enable dynamic personalization approaches that improve the accessibility of language technologies for underserved communities, in which collecting sufficient training data is physically impossible.
Bio: Hej! I’m a postdoc at the IT University of Copenhagen's NLPnorth Lab and the Danish Pioneer Centre for Artificial Intelligence, working with Anna Rogers. My research centers around identifying and leveraging consistencies in the learning dynamics of language models in order to make their training more efficient. On the data side, we’re looking into how linguistic properties in the training data lead to different generalization capabilities. On the modeling side, we investigate how different types of knowledge are represented across pre-training. We’ve applied findings from both pillars to make model adaptation to low-resource scenarios more efficient: e.g., improving cultural alignment of LMs to Danish, and enabling speech recognition for people with speech disabilities.
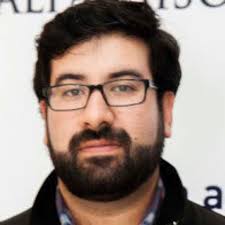
Guest Talk: Context-Aware Retrieval Augmented Generation Framework - 12.03.2025 10:00
Speaker: Dr. Héctor Allende-Cid (Fraunhofer IAIS)
Abstract: In this talk, I will present CARAG, a Context-Aware Retrieval Augmented Generation framework that improves Automated Fact Verification (AFV) by incorporating both local and global explanations. Unlike traditional fact-checking methods that focus on isolated claims, CARAG leverages thematic embedding aggregation to verify claims in a broader contextual landscape. I will also introduce CARAG-u, an unsupervised extension that eliminates the need for predefined thematic annotations, dynamically deriving contextually relevant evidence clusters from unstructured data. CARAG-u maintains strong performance while increasing adaptability and scalability. Through benchmarks on the FactVer dataset, I will demonstrate how these frameworks enhance explainability and thematic coherence, advancing the role of AI in trustworthy, transparent fact verification.
Bio: Dr. Héctor Allende-Cid is a Senior Researcher in the Natural Language Understanding Group at Fraunhofer IAIS since August 2023. He holds an Computer Science Engineering degree (2007), a Master (2009) and Doctorante in Computer Science (2015) from Universidad Técnica Federico Santa María, Chile, and has been a Full Professor at Pontificia Universidad Católica de Valparaíso since 2015. He served as President of the Chilean Pattern Recognition Association from 2017 until 2021. His research interests include NLP, Machine Learning, Time Series Forecasting, and Computer Vision.
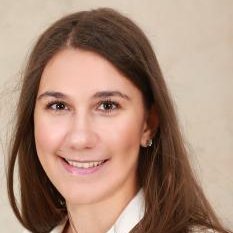
FEBRUARY 2025
Guest Talk: TheAItre and the Challenges of NLG Evaluation - 5.2.2025 10:00
Speaker: Patrícia Schmidtová (Charles University)
Patricia is one of the faces behind TheAItre https://www.theaitre.com/, the LLM project where she generated scripts that real actors performed as a regular theater play in Prague with numerous successful repetitions:https://www.youtube.com/watch?v=8ho5sXiDX_A , back then even with GPT-2 !
Nowadays her research mainly focuses on LLM benchmaking and evaluating the quality of generated text:https://aclanthology.org/2024.eacl-long.5/
Patrícia's talk will therefore cover these two topics:
1) Theatre Play Script Generation with GPT
In the first part of my talk, I will discuss the joys and challenges of my master's research on generating the script of a full-length play using GPT-2. Namely, I will share some of the strategies we used to navigate around the limited context length of the model, getting the characters to have a consistent persona, and above everything else, making the play interesting to watch for the audience.
2) Data Contamination and Other Challenges of NLG Evaluation
In the second part, I will share my ongoing doctoral research on evaluating natural language generation. I will discuss our work on data contamination, present an overview of how NLG is evaluated across different specific tasks, and share my challenges of evaluating the semantic accuracy of summarization at a scale when no reference is available.
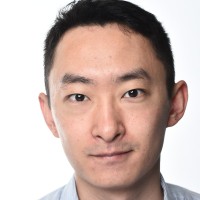
JANUARY 2025
Guest Talk: AI Agents From Foundation to Application - 24.1.2025 10:00
Speaker: Dr. Yunpu Ma (LMU)
Abstract: In this lecture, we will journey through the core principles of AI agents, building a conceptual bridge from foundational theories to cutting-edge practical implementations. Attendees will gain insights into how autonomous agents operate, starting with basic AI agent architectures and evolving into sophisticated web automation systems. Highlighting our latest research with WebPilot, the lecture will showcase how integrating Monte Carlo Tree Search with a dual optimization strategy addresses the complexities of dynamic web tasks—mitigating vast action spaces and uncertainty through strategic exploration and adaptive decision-making.
Bio: Dr. Yunpu Ma is a Postdoc at the University of Munich, working with Prof. Volker Tresp and Prof. Thomas Seidl on multimodal foundation models and dynamic graphs. Additionally, he is a research scientist at Siemens, specializing in quantum machine learning. Before Siemens, he spent three years as an AI researcher at LMU, where he earned his Ph.D., focusing on temporal knowledge graphs. His research interests encompass structured data learning, multimodal foundation models, and quantum machine learning. His ultimate research goal is to advance general AI.
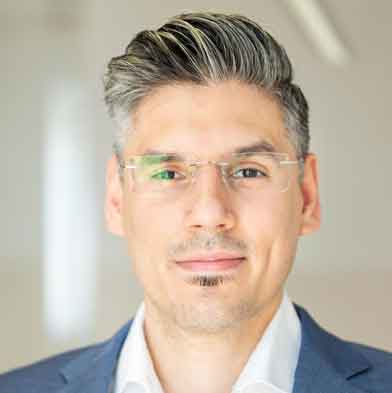
Guest Talk: How To Train A Multilingual Large Language Model? - 9.1.2025 10:00
Speaker: Dr. Mehdi Ali (Fraunhofer IAIS)
The Teuken 7B model, a large language model for *European languages*, has recently made the news. If you’re interested in knowing how such models are trained, this week’s speaker is one of the lead scientists who’s done it.
As part of the Lamarr NLP monthly meetings, this week we have the pleasure to host Dr. Mehdi Ali from the Fraunhofer IAIS who will give a guest lecture on How To Train A Multilingual Large Language Model?.
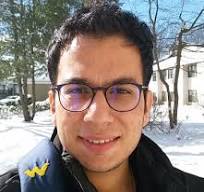
DECEMBER 2024
Guest Talk: Reliable Evaluation of Interactive LLM Agents in a World of Apps and People: AppWorld - 11.12.2024 10:00
Speaker: Harsh Trivedi (Stony Brook University)
Tomorrow in the Lamarr NLP Colloquium we have the pleasure to host Harsh Trivedi from Stony Brook University. His recent work, AppWorld, received a Best Resource Paper award at ACL’24, and his work on AI safety via debate received a Best Paper award at the ML Safety workshop at NeurIPS’22. His work has made waves at Stanford, Google, Apple, and many other places (https://appworld.dev/talks)
Abstract: We envision a world where AI agents (assistants) are widely used for complex tasks in our digital and physical worlds and are broadly integrated into our society. To move towards such a future, we need an environment for a robust evaluation of agents' capability, reliability, and trustworthiness.
In this talk, I'll introduce AppWorld, which is a step towards this goal in the context of day-to-day digital tasks. AppWorld is a high-fidelity simulated world of people and their digital activities on nine apps like Amazon, Gmail, and Venmo. On top of this fully controllable world, we build a benchmark of complex day-to-day tasks such as splitting Venmo bills with roommates, which agents have to solve via interactive coding and API calls. One of the fundamental challenges with complex tasks lies in accounting for different ways in which the tasks can be completed. I will describe how we address this challenge using a reliable and programmatic evaluation framework. Our benchmarking evaluations show that even the best LLMs, like GPT-4o, can only solve ~30% of such tasks, highlighting the challenging nature of the AppWorld benchmark.I will conclude by laying out future research that can be conducted on the foundation of AppWorld, such as the evaluation and development of multimodal, collaborative, safe, socially intelligent, resourceful, and fail-tolerant agents that can plan, adapt, and learn from environment feedback.
Project Website:
<https://appworld.dev/>https://appworld.dev/
Bio: Harsh Trivedi is a final year PhD researcher at Stony Brook University, advised by Niranjan Balasubramanian. He is broadly interested in the development of reliable, explainable AI systems and their rigorous evaluation. Specifically, his research spans the domains of AI agents, multi-step reasoning, AI safety, and efficient NLP. He has interned at AI2 and was a visiting researcher at NYU. If you're interested, you can get in touch with him at hjtrivedi@cs.stonybrook.edu for follow-ups.
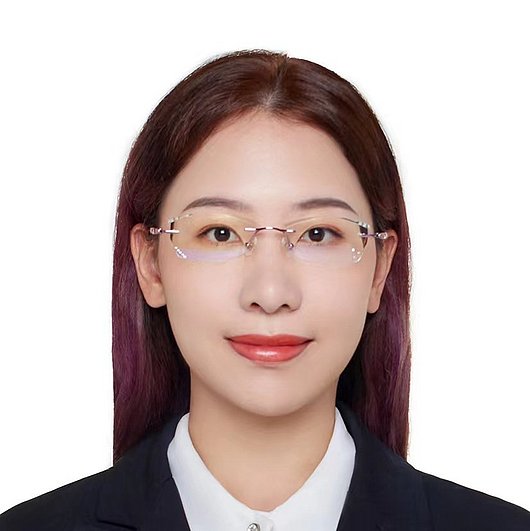
OCTOBER 2024
Guest Talk: Understanding and Reasoning in Structured and Symbolic Representations. - 9.10.2024 10:00
Speaker: Tianyi Zhang (University of Pennsylvania)
Abstract: This talk outlines my research trajectory in language understanding and reasoning. I begin with event extraction through question-answering techniques, followed by constructing event schemas. Subsequently, I investigate the translation of natural language into symbolic representations to facilitate faithful reasoning. Currently, my work explores training language models using both natural language and knowledge graphs, as well as evaluating narratives through knowledge graphs.
Bio: Tianyi Zhang is a visiting scholar at the University of Bonn, supervised by Prof. Lucie Flek. Her research focuses on extracting entity-relationship knowledge from text and reasoning with structured and symbolic representations. She received master's degrees in Data Science and in Learning Science and Technology from the University of Pennsylvania.
September 2024
Prof. David Jurgens (University of Michigan) >> Lamarr Conference Talk "Do Foundation Models have Personalities? The Risks and Opportunities for Model Aligment and Personification
August 2024
Prof. Paolo Rosso (Universitat Politècnica de València) >> "1:1s Deep Dives with the Data Science and Language Technologies on Fake News
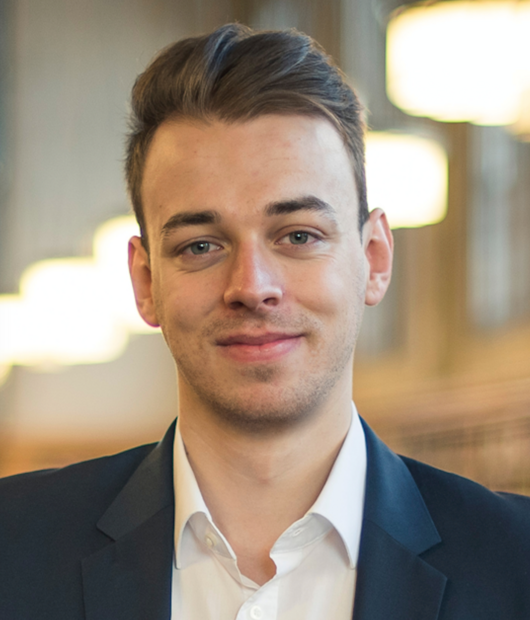
AUGUST 2024
Guest Talk: Trustworthy Machine Learning for AI Safety and AI-driven Scientific Discovery - 14.8.2024 10-11
Speaker: Dr. Tim G. Rudner (NYU Data Science)
Abstract: Machine learning models, while effective in controlled environments, can fail catastrophically when exposed to unexpected conditions upon deployment. This lack of robustness, well-documented even in state-of-the-art models, can lead to severe harm in high-stakes, safety-critical application domains such as healthcare and to bias and inefficiencies in AI-driven scientific discovery. This shortcoming raises a central question: How can we develop machine learning models we can trust?
In this talk, I will approach this question from a probabilistic perspective, stepping through ways to address deficiencies in trustworthiness that arise in model training and model deployment. First, I will demonstrate how to improve the trustworthiness of neural networks used in medical imaging by incorporating data-driven, domain-informed prior distributions over model parameters into neural network training. Next, I will show how a probabilistic perspective on prediction can make vision-language models used in healthcare settings more human-interpretable and transparent. Throughout this talk, I will highlight carefully designed evaluation procedures for assessing the trustworthiness of machine learning models used in healthcare and AI-driven scientific discovery.
Bio: Tim G. J. Rudner is a Data Science Assistant Professor and Faculty Fellow at New York University’s Center for Data Science and an AI Fellow at Georgetown University's Center for Security and Emerging Technology. He conducted PhD research on probabilistic machine learning at the University of Oxford, where he was advised by Yee Whye Teh and Yarin Gal. The goal of his research is to create trustworthy machine learning models by developing methods and theoretical insights that improve the reliability, safety, transparency, and fairness of machine learning systems deployed in safety-critical and high-stakes settings. Tim holds a master’s degree in statistics from the University of Oxford and an undergraduate degree in applied mathematics and economics from Yale University. He is also a Qualcomm Innovation Fellow and a Rhodes Scholar.
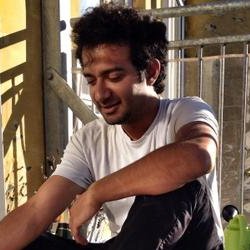
JULY 2024
Guest Talk: Diagnosing NLP: Sources of Social Harms of NLP - 24.7.2024 14:00
Speaker: Dr. Zeerak Talat (MBZUAI / Edinburgh University)
Abstract: The advances in language technologies has seen attempts at addressing increasingly complex tasks such as hate speech detection, in addition to longstanding tasks such as language generation and summarization. However, in spite of the advances and increased public and research attention to such tasks, language technologies broadly still broadly and widely cause social harms such as the propagation of social biases (in increasingly sensitive areas). In this talk, I will discuss sources of biases and suggested technical interventions, in order to identity whether they address the underlying issues. In particular, I will attend to the political reality of how language technologies are deployed and what their use is. Through this discussion, I hope to highlight pathways for research on language technologies to be used in service of society.
Bio: Zeerak Talat's research seeks to, on one hand, examine how machine learning systems interact with our societies and the downstream effects of introducing machine learning to our society; and on the other, to develop tools for content moderation technologies to facilitate open democratic dialogue in online spaces. Zeerak is an incoming Chancellor's Fellow (~Assistant/Junior Professor) at the Edinburgh Centre for Technomoral Futures and Informatics at Edinburgh University. They are currently a research fellow at Mohamed Bin Zayed University of Artificial Intelligence and a visiting research fellow at the Alexander von Humboldt Institute for Internet and Society. Prior to this, Zeerak was a post-doctoral fellow at the Digital Democracies Institute at Simon Fraser University, and received their Ph.D. in computer science from the University of Sheffield.
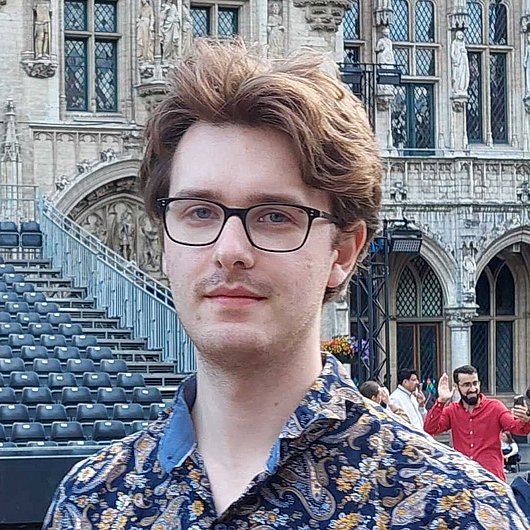
APRIL 2024
Guest Talk: MuZero - Dynamic Learning for LLM Dialog Planning - 30.4. 13:30-14:15
Speaker: David Kaczer (KU Leuven)
Abstract: While large language models (LLMs) perform well on a variety of language-related tasks, they struggle with tasks that require planning. We apply the existing MuZero algorithm to enhance the planning capabilities of LLMs in dialog settings. MuZero uses a neural network to represent observations into a latent space, and then performs Monte Carlo tree search in the latent space using dynamics learned through self-play. We develop a simulated dialog environment to train the MuZero-based model on conversations with a generative LLM such as DialoGPT. We also investigate modifications to the model architecture, such as replacing the representation network by a transformer pretrained on sentence classification. We evaluate our algorithm on realistic multi-turn dialog planning tasks, such as steering the dialog topic to a predefined goal.
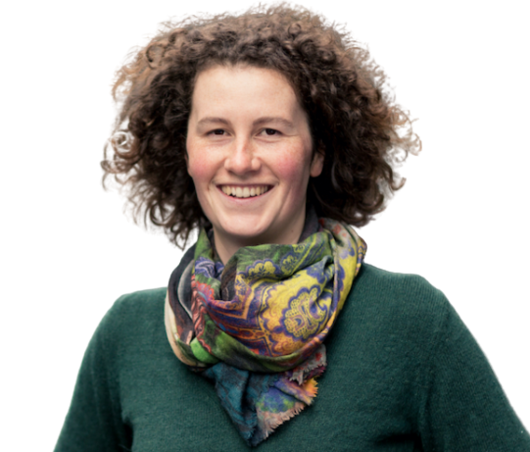
OCTOBER 2023
Guest Talk: Aligning existing information-seeking processes with Conversational Information Seeking And much more
Speaker: Dr. Johanne Trippas (RMIT) - 25.10.2023 15:00
Abstract: This talk explores the theoretical aspects of Conversational Information Seeking (CIS) while combining ongoing interaction log analysis and envisioning future research. This talk begins with the core theories underpinning CIS, providing a foundation for the practical insights that follow. The presentation then explores real-world user engagements through interaction log analysis, revealing key patterns and behaviours. The focus shifts to the horizon of information retrieval, with innovative concepts in immersive information seeking. These visionary ideas represent the future of knowledge access.
Bio: Johanne Trippas is a Vice-Chancellor's Research Fellow at RMIT University, specializing in Intelligent Systems, focusing on digital assistants and conversational information seeking. Her research aims to enhance information accessibility through conversational systems, interactive information retrieval, and human-computer interaction. Additionally, Johanne is currently part of the National Institute of Standards and Technology (NIST) Text REtrieval Conference (TREC) program committee and is an ACM Conference on Human Information Interaction and Retrieval (CHIIR) steering committee member. She serves as vice-chair of the SIGIR Artifact Evaluation Committee, tutorial chair for the European Conference on Information Retrieval (ECIR) '24, general chair of the ACM Conversational User Interfaces (CUI) '24, and ACM SIGIR Conference on Information Retrieval in the Asia Pacific (SIGIR-AP) '23 proceedings chair.
Lamarr NLP Guest Talk: Dr. Florian Mai (KU Leuven)
Lamarr NLP Guest Talk: Dr. Cass Zhao (Uni Sheffield)
EVENTS, where you can meet us
2025
- INLG 2025, The 18th International Natural Language Generation Conference, Hanoi, Vietnam, October 29 - November 2, 2025 with Program Co-Chair Prof. Dr. Lucie Flek >> CALLS (Deadline July 15, 2025)
- Finale des Bundeswettbewerbs Künstliche Intelligenz, Frankfurt, Germany, November 14, 2025, Member of the Jury: Prof. Dr. Lucie Flek
- ECML 2025, European Conference on Machine Learning and Principles and Practice of Knowledge Discovery in Databases, Porto, Portugal, September 15 - 19, 2025 >> CHALLENGE: Colliding with Adversaries (till June 23, 2025) organized by Prof. Lucie Flek, Prof. Matthias Schott, Dr. Akbar Karimi, Timo Saalo
- ACL 2025, The 63rd Annual Meeting of the Association for Computational Linguistics, Vienna, Austria, July 27 - August 1st, 2025
- ICWE 2025, 25th International Conference on Web Engineering, Delft, Netherlands, June 30 - July 03, 2025, Keynote by Prof. Dr. Lucie Flek - Can Language Models Be Truly Human-Centered? Rethinking Personalization, Empathy, and Social Intelligence
- HealTAC 2025, 8th Healthcare Text Analytics Conference, Glasgow, Scotland, June 16 - 18, 2025
- SeWeBMeDA-2025, 8th Workshop on Semantic Web Solutions for Large-Scale Biomedical Data Analytics, Portoroz, Slovenia, June 1, 2025
- International Conference on Large-Scale AI Risks, Institute of Philosophy of KU Leuven, Belgium, May 26 - 28, 2025 co-organized by Dr. Florian Mai (Junior Group Leader in Data Science and Language Technologies Group, University of Bonn)
- ICLR 2025, The Thirteenth International Conference on Learning Representations, Singapore EXPO, April 24 - 28, 2025
- 3rd HumanCLAIM Workshop, The Human Perspective on Cross-Lingual AI Models, Göttingen, March 26 - 27, 2025
- BMBF-VA Weltfrauentag 2025, Berlin, March 7, 2025
- Responsible AI in Action, Berlin, March 6, 2025
- The 39th Annual AAAI Conference on Artificial Intelligence, Philadelphia, Pennsylvania, USA, February 25 - March 4, 2025
- Lamarr Lab Visits: 2025.1, Dortmund, February 18 - 20, 2025
2024
- The Workshop on Computational Linguistics and Clinical Psychology, Malta, Thursday March 21, 2024
- Transatlantic AI Symposium: Europe's Heartbeat of Innovation, San Francisco, California, April 17, 2024
- Machine Learning Prague 2024, April 22 – 24, 2024
- LREC-COLING 2024, Lingotto Conference Centre – Torino, May 20 – 25, 2024
- DataNinja sAIOnARA 2024 Conference, Bielefeld University, June 25 - 27, 2024
- The ACM Conversational User Interfaces 2024, Luxembourg, July 8 - 10, 2024
- Human-Centered Large Language Modeling Workshop, ACL 2024, Bangkok, August 15, 2024
- AI24 - The Lamarr Conference featuring industrial AI, Dortmund, September 4 - 5, 2024
- Next Generation Environment for Interoperable Data Analysis - 2nd Expert Workshop, Dortmund, September 17 - 18, 2024
- Scaling AI Assessments - Tools, Ecosystems and Business Models (Zertifizierte KI), Cologne, September 30 - October 01, 2024
- KI Forum NRW 2024, Cologne, October 10, 2024
- The 2024 Conference on Empirical Methods in Natural Language Processing, Miami, Floria, November 12 - 16, 2024
- NeurIPS 2024, Vancouver, December 10 - 15, 2024
...happy to meet you anywhere around the world and anytime in Bonn, Germany