Large language models for drug discovery
New study conducted by Prof. Dr. Bajorath and Sanjana Srinivasan at b-it and the Lamarr-Institute at the University of Bonn show the potential of language models in finding new medications. The researchers have created a chemical language model comparable to ChatGPT to predict potential active ingredients with special properties. Following a training phase, the AI was able to exactly reproduce the chemical structures of compounds with known dual-target activity that may be particularly effective medications.
Nowadays, a short prompt in ChatGPT is all it takes to create birthday poetry or nursery rhymes, and within a few seconds the AI spits out a long list of words that rhyme with the (birthday) child’s name. It can even produce a sonnet to go with it if you like.
Researchers from the Bonn-Aachen International Center for Information Technology at the University of Bonn have developed a comparable model in their study, termed a chemical language model. Unlike the poetic application, this model does not create rhymes; instead, it illustrates the structural formulas of chemical compounds that possess a notable characteristic: the ability to bind to two different target proteins simultaneously. This dual binding capability can inhibit two enzymes within an organism.
Finding active ingredients with a double effect
There is a strong demand for active ingredients that exhibit this dual effect. Prof. Dr. Jürgen Bajorath, head of b-it's research group Life Science Informatics and Data Science as well as Area Chair Life Sciences at the Lamarr Institute for Machine Learning and Artificial Intelligence at Uni Bonn, emphasizes the significance of such compounds in pharmaceutical research due to their polypharmacological properties. “Because compounds with desirable multi-target activity influence several intracellular processes and signaling pathways at the same time, they are often particularly effective – such as in the fight against cancer.” In principle, this effect can also be achieved by co-administration of different drugs. However, there is a risk of unwanted drug-drug interactions and different compounds are also often broken down at different rates in the body, making it difficult to administer them together.
Identifying a molecule that specifically targets a single protein is challenging, and designing compounds with predetermined dual effects is even more complex. Chemical language models may offer assistance in this area moving forward. Similar to how ChatGPT learns from vast amounts of text, these models are trained on smaller datasets but are still fed textual representations like SMILES strings that depict organic molecules and their structures through sequences of letters and symbols. “We have now trained our chemical language model with pairs of strings,” says Sanjana Srinivasan from Bajorath’s research group. “One of the strings described a molecule that we know only acts against one target protein. The other represented a compound that, in addition to this protein, also influences a second target protein.”
Learning chemical connections and inspiring innovative thinking
The model learned from over 70,000 such pairs, allowing it to understand the differences between standard active compounds and those with dual effects. When provided with a compound targeting one protein, it could suggest other molecules that would also act against an additional target.
Typically, compounds with dual effects target similar proteins performing analogous functions. However, researchers are also interested in finding active ingredients that affect entirely different classes of enzymes or receptors. To prepare the AI for this broader task, fine-tuning was conducted after the initial training phase using specialized pairs to guide the algorithm on which protein classes the suggested compounds should target.
Following this refinement process, the model successfully generated molecules known to act on the desired combinations of target proteins. “This shows that the process works,” says Bajorath. In his opinion, however, the strength of the approach is not that new compounds exceeding the effect of available pharmaceuticals can immediately be found. “It is more interesting, from my point of view, that the AI often suggests chemical structures that most chemists would not even think of right away,” he explains. “To a certain extent, it triggers ‘out of the box’ ideas and comes up with original solutions that can lead to new design hypotheses and approaches.”
More Information
Publication: Sanjana Srinivasan and Jürgen Bajorath: Generation of Dual-Target Compounds Using a Transformer Chemical Language Model; Cell Reports Physical Science; DOI: 10.1016/j.xcrp.2024.102255; https://doi.org/10.1016/j.xcrp.2024.102255
To the original press release: https://www.uni-bonn.de/en/news/207-2024
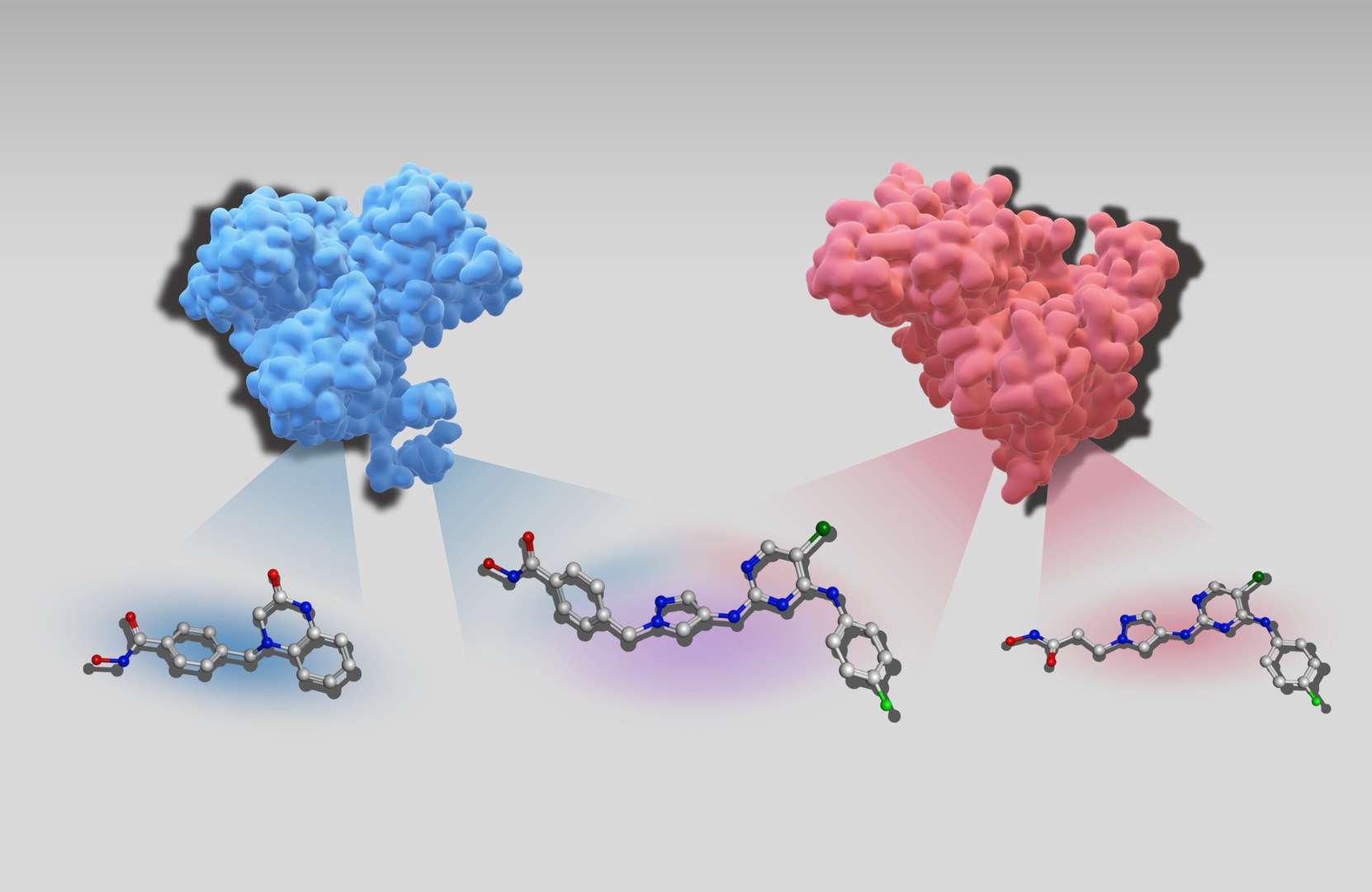