How large language models affect social collaboration and why we need to shape it
A recent article published in "Nature Human Behaviour" explores the potential impact of large language models (LLMs) on collective intelligence and societal decision-making. The study, led by researchers from Copenhagen Business School and the Max Planck Institute for Human Development in Berlin, brings together insights from 28 scientists across various disciplines. Among the authors is also Prof. Dr. Lucie Flek, Head of b-it's Data Science and Language Technologies group. The study provides internal recommendations for researchers and policy makers to ensure that LLMs complement rather than detract from the benefits of collective intelligence.
The advent of LLM-powered platforms such as ChatGPT has brought AI into the mainstream. These AI systems, which analyze and generate text using vast datasets and advanced learning techniques, offer both promising opportunities and significant risks for collective intelligence.
Enhancing accessibility, collaboration, and idea generation
One of the key benefits highlighted in the study is the potential for LLMs to increase accessibility in collective processes. By providing translation services and writing assistance, these models can break down barriers and enable more diverse participation in discussions. LLMs can also help with forming opinions by sharing information, summarizing different viewpoints, and finding consensus among diverse perspectives.
Undermining knowledge commons, false consensus and marginalization
Despite their benefits, the researchers warn of several risks associated with the widespread use of LLMs. There's a concern that LLMs could reduce people's motivation to contribute to collective knowledge platforms like Wikipedia and Stack Overflow. This shift towards reliance on proprietary models may endanger the openness and diversity of the knowledge landscape. Jason Burton, the lead author of the study, points out a critical issue: "Since LLMs learn from information available online, there is a risk that minority viewpoints are unrepresented in LLM-generated responses. This can create a false sense of agreement and marginalize some perspectives"
Recommendations for responsible development
To ensure that large language models enhance rather than undermine human collective intelligence, the researchers propose several recommendations. First, LLM developers should disclose the sources of their training data to promote enhanced transparency. Additionally, they suggest implementing external audits that establish monitoring systems to better understand LLM development and mitigate any potential negative effects. Finally, the researchers emphasize the importance of addressing goals related to diverse representation in both the development and training processes of LLMs.
Future research directions
The researchers propose several key questions for future research to explore how large language models can be leveraged to enhance collective intelligence while mitigating potential risks. They emphasize the need to investigate strategies for maintaining diverse perspectives, including those of minority groups, and preserving functional diversity in human-LLM interactions. Additionally, they highlight the importance of addressing issues related to credit attribution and accountability when collective outcomes involve collaboration with LLMs.
Co-author of the article, Prof. Dr. Lucie Flek, heads the research group Data Science & Language Technologies at b-it. Her research on natural language processing focuses on three main areas: personalization and alignment, knowledge augmentation, and robustness, fairness, and efficiency. Her team develops algorithms to enhance user experiences tailored to individual needs, improves knowledge systems through better data integration and semantic understanding, and addresses challenges related to bias and efficiency in data-driven technologies. Through interdisciplinary collaboration and innovative methodologies, they aim to tackle real-world problems and invite inquiries from those interested in advancing these fields.
As LLMs continue to shape our information landscape, the comprehensive study featured in Nature Human Behaviour provides crucial insights for researchers, policymakers, and developers. By proactively addressing these challenges and opportunities, we can work towards harnessing the power of LLMs to create a smarter and more inclusive society.
Key points in a nutshell
- LLMs are changing how people search for, use, and communicate information, which can affect the collective intelligence of teams and society at large.
- LLMs offer new opportunities for collective intelligence, such as support for deliberative, opinion-forming processes, but also pose risks, such as endangering the diversity of the information landscape.
- If LLMs are to support rather than undermine collective intelligence, the technical details of the models must be disclosed, and monitoring mechanisms must be implemented.
More Information
Original publication
Burton, J. W., Lopez-Lopez, E., Hechtlinger, S., Rahwan, Z., Aeschbach, S., Bakker, M. A., Becker, J. A., Berditchevskaia, A., Berger, J., Brinkmann, L., Flek, L., Herzog, S. M., Huang, S. S., Kapoor, S., Narayanan, A., Nussberger, A.-M., Yasseri, T., Nickl, P., Almaatouq, A., Hahn, U., Kurvers, R. H., Leavy, S., Rahwan, I., Siddarth, D., Siu, A., Woolley, A. W., Wulff, D. U., & Hertwig, R. (2024). How large language models can reshape collective intelligence. Nature Human Behaviour. Advance online publication. https://www.nature.com/articles/s41562-024-01959-9
Article in Nature Human Behaviour:https://www.nature.com/articles/s41562-024-01959-9
Press release by Max Planck Institute:https://www.mpib-berlin.mpg.de/press-releases/llms-and-collective-intelligence
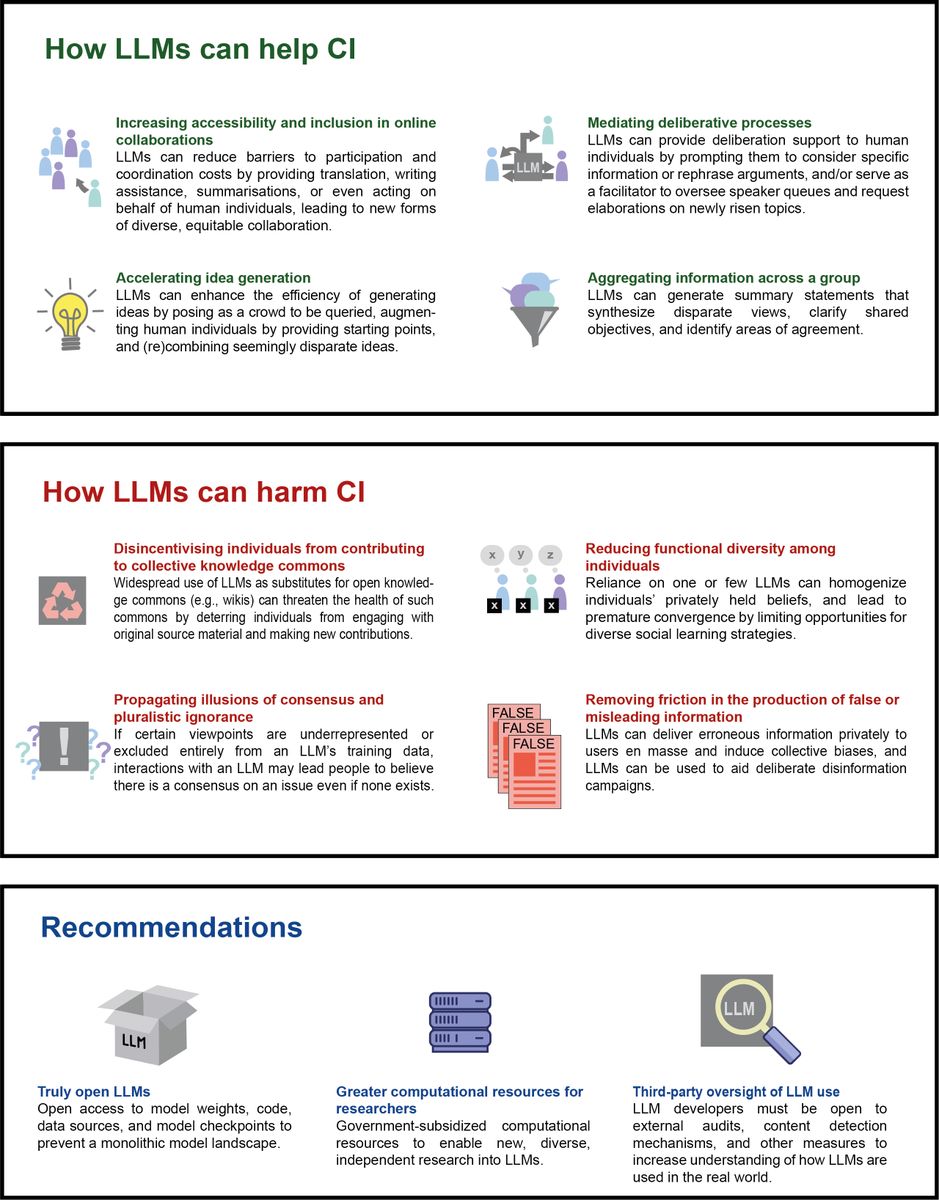