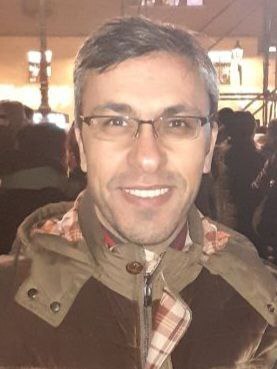
Dr. Akbar Karimi
While language models are becoming better and better every day, they still have vulnerabilities to real-world errors and adversarial players and in many cases, there’s still lack of proper data in order for these models to perform well on newly designed tasks. As a result, among other topics in NLP, my focus is on improving robustness of language models to a variety of changes.
In pursuit of more robust language models, I’ve worked on adversarial and simple data augmentation methods. In the former, artificial adversarial data is created in the embedding space and in the latter, noise is injected into the raw input data. These methods have helped models become more immune to such changes, hence improving their ability to recognize user sentiments and more accurately identify what they’re talking about in services and products reviews.
Recent generative models, although better than their previous counterparts in many areas, have also shown problems such as hallucination, bias and poor reasoning capabilities. Studying these issues are also at the center of my research efforts.
Areas of Interest
- Robustness
- Large language models
- Data augmentation methods
- Adversarial attacks and defenses
- Explainability and interpretability methods